Coaching Strategies for Leaders in Data, Analytics, and AI
Written on
Chapter 1: Introduction to Coaching in Data and AI
If you're at the helm of teams focused on Data, Analytics, Data Science, or AI, this blog series is tailored for you! Here, we delve into principles and effective practices that have proven beneficial during my tenure leading teams in Data Engineering, Analytics, and AI across both startups and established companies.
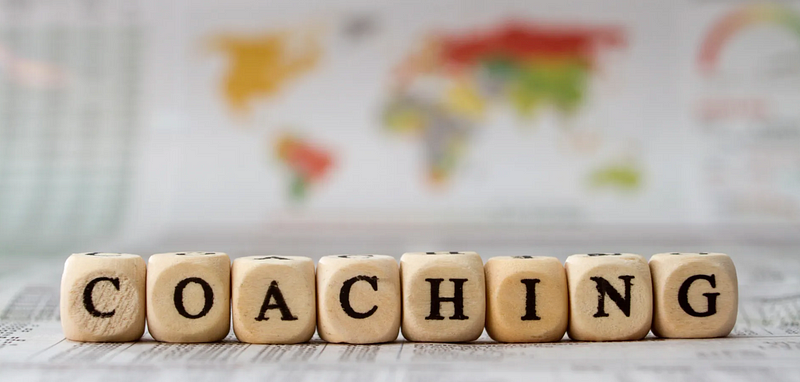
1. Embrace Innovation through Tool Experimentation
The data science landscape is constantly changing, and the relevance of tools can differ based on your organization’s maturity in data and analytics. It's vital to regularly experiment with new technologies and methodologies instead of relying solely on traditional, internally developed solutions. Assign clear responsibilities for investigating, testing, and integrating new tools and processes to enhance your maturity trajectory.
2. Recognize Different Data Engagement Levels
Within your organization, business teams exhibit varying degrees of engagement with data, classified as data-informed, data-inspired, or data-driven. Understanding these differences is crucial for fostering effective collaboration. A data-informed team aims to grasp current and historical performance, while a data-inspired team seeks to identify trends and insights for strategic enhancement. Conversely, a data-driven team leverages data to answer specific questions and derive actionable insights.
3. Allocate Resources for Significant Decisions
Analytics teams play a pivotal role in business decision-making. Decisions can range in complexity and impact, so it's essential to ensure that high-stakes initiatives are not executed as last-minute tasks or under unrealistic deadlines by the analytics team.
4. Optimize Insight Maintenance Efforts
Data analytics teams primarily engage in two types of work: maintaining operational dashboards and conducting exploratory data analysis to uncover business growth opportunities. It's important to identify instances where excessive time is spent on dashboard maintenance due to issues like poor data quality, inadequate change management, and ambiguous business processes.
5. Develop a Clear Collaboration Framework
Is there a defined collaboration framework between business teams and your analytics/science team? If not, the approach may devolve into a chaotic mix of individual experiences from team members based on their previous roles. To avoid this disorganization, proactively develop and communicate a clear collaboration model.
6. Create a Communication Strategy for Production Challenges
Do you have a standardized communication strategy for your teams to follow during production model issues, dashboard problems, or experiments? Teams often waste time crafting custom communication documents, impacting productivity. Instead, establish a streamlined communication template with designated responsibilities, empowering teams closest to the issue to report and share updates directly.
7. Enhance Understanding of Data Details
Data Analysts, Scientists, and AI developers often spend considerable time grasping the intricacies of data—what is included, what is excluded, data collection assumptions, and the differences between similar attributes across datasets. While improved data documentation is beneficial, it may not always be prioritized. Set up regular meetings with data source teams to clarify questions regarding data nuances, as those closest to the data have the deepest understanding of these complexities, preventing errors in insight generation.
8. Foster Operational Excellence Across Teams
Data analytics, science, and AI teams frequently devote significant time to resolving post-production issues. Make operational excellence a priority across all teams, not just within DataOps/MLOps. Provide training on operational excellence skills, such as developing better error handling mechanisms, generating anomaly alerts, and implementing data quality checks in models and dashboards.
9. Streamline Timeline Expectations with Business Partners
Data Analytics leaders often encounter difficulties establishing realistic timelines with their business counterparts. To effectively manage these expectations, assess aspects such as data availability and dependencies on source teams that may need prioritization. Additionally, it’s crucial to align on the accuracy level required for decision-making, as more general insights can often be delivered more swiftly.
10. Build a Decision Repository
Analytics teams collaborate with business partners on various decisions. Establishing a centralized repository for documenting all decisions—big or small—contributed by the analytics team is invaluable. Without such an inventory, it becomes challenging to measure and optimize the impact of decisions and the ROI that the analytics team delivers.
Chapter 2: Further Coaching Principles for Data Leaders
If you found this information useful, consider following me on LinkedIn or Medium for additional insights and coaching patterns.